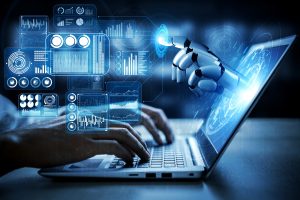
Artificial intelligence (AI) has gone from a sci-fi television buzzword to an instrument we all encounter and interact with in daily life. With this evolution comes opportunities to develop AI systems solutions for a wide range of problems.
In a recently published review in the Nature Partner Journal , Johns Hopkins computer scientists discuss the need for centering the development of explainable AI systems on the users who later are going to utilize them.
Explainable AI models are when users can easily and readily understand the algorithm’s reasoning behind its predictions and decisions. The widely popular assumption is that the more interpretable, or understandable, the system, the more trust a user can give the machine. However, as research shows, this is not always true because people, their context, and their minds are complex.
Authors of the commentary are Mathias Unberath, assistant professor of computer science; John C. Malone Assistant Professor of Computer Science, Chien-Ming Huang; and computer science graduate students Catalina Gomez and Haomin Chen.
Appreciating a recommendation for a new song are low-stakes tasks; any mistake of the AI system does not cause harm, besides, perhaps, some minor loss of time. But in other scenarios – for example in finance, justice, or healthcare – involving AI in decision-making can have dire consequence and may call for information beyond the recommendation: explanations of how the AI system reached the conclusion.
However, not all explanations are equally useful for all users, cautions Unberath. This is because people differ in their prior knowledge of the subject matter, context in which the AI is being used, or expectations on the system and its explanations. Thus, over-eager development of explainable AI systems without considering the user and their needs is likely to result in systems that are unintelligible by the target users, and thus, ultimately useless.
The researchers call for AI developers to explicitly acknowledge the audience that will later use the AI system and design the algorithm directly for their needs. In short, these systems cannot be developed without the user in the focus of all design decisions.
But it does not stop there. Even more importantly, the hypotheses that inform the AI system’s design must be evaluated with the targeted clients in mind, to make sure that the solution achieves the envisioned goals. These tests, with users in the loop, are largely absent from the current literature especially in the healthcare domain, says Unberath.
And busy Healthcare providers may not have time to run a user-study on a new algorithm. However, finding solutions for how to better test AI algorithms, explainable or not, with humans-in-the-loop is going to be a critical step in ensuring the adoption of AI in healthcare and other industries.
AI can power self-driving cars, suggest parole decisions, approve loans, and contribute to other high-stakes decision making tasks. But these developments must stem from a clear understanding of the desirable goal, both in terms of the algorithm itself but also regarding its usability, user reliance, trust, agency, and other human factors.
“Less than half of these developed AI systems are not specific about who they are created for and who the users are. This paper is an appeal to the AI development community to be explicit about who the AI system is for and what their needs are to individualize the development,” says Unberath.
Unberath and Huang address these topics in their teaching at the JHU Department of Computer Science, through their classes such as Human-Computer Interaction, Interpretable Machine Learning Design and Artificial Intelligence Systems Design.
To read their full publication on translational AI systems, click here.