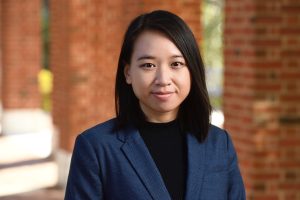
Anqi (Angie) Liu joins Johns Hopkins University as an assistant professor of computer science. She is affiliated with the Johns Hopkins Mathematical Institute for Data Science (MINDS) and the Johns Hopkins Institute for Assured Autonomy (IAA).
Liu received her PhD from the University of Illinois at Chicago. Before joining Johns Hopkins, she was a postdoctoral fellow in the Department of Computing and Mathematical Sciences at California Institute of Technology.
Tell us a little bit about your research.
My research interest lies in machine learning for trustworthy AI. I’m interested in developing principled machine learning algorithms for building more reliable, trustworthy, and human-compatible AI systems in the real world. This requires the machine learning algorithms to be robust to the changing data distributions and environments, to provide accurate and honest uncertainty estimates, and to consider human preferences and values in the interaction.
I’m particularly interested in high-stake applications that concern the safety and societal impact of AI. A few of my current projects include uncertainty estimation and calibration under distribution shift, learning from human-generated ambiguous data, and human-AI decision-making under uncertainty.
Tell us about a project you are excited about.
I am very excited about my projects on the direction of uncertainty estimation under distribution shift. Distribution shift is notoriously ubiquitous and harmful for most machine learning algorithms. It happens when the training data is biasedly sampled, noisy, incomplete, and thus the training distribution does not match the distribution of the target domain. In particular, we are focusing a special case of distribution shift: covariate shift, when the conditional label distribution is shared between source and target distributions while the marginal input distribution shifts. It is prevalent in practice: for example, we may want to study the voting behavior in the DC area but only have access to polling data collected from the Chicago area. Even though the voting behavior of all subpopulations is similar between Chicago and DC, given the sample selection bias (let’s say Chicago has a larger Asian population), machine learning models will not generalize well outside of the training distribution. Also, most uncertainty estimation methods lose their effectiveness or guarantees under distribution shift.
Therefore, we develop robust learning methods and uncertainty quantifiers for predictors under distribution shift. We also apply our methods to benefit downstream tasks like invariant and fair learning from multiple domains/subpopulations, active learning, model auditing and calibration, safe control and decision-making, and human-AI collaboration.
Why this? What drives your passion for your field?
My passion for this work comes from my frustration when experiencing and observing failure cases of the current ML/AI systems. These failures can cause further issues after propagating and aggregating in the feedback loop of the human/society-AI interactions.
Making AI more robust, safer, and less biased – even under imperfect data and training environments – is crucial when our systems become larger and faster. And this is especially true if the system is human-facing; making it understandable and considerate of human values will hopefully prevent catastrophic risks. This is a hard but essential research problem that will influence many aspects of society, as AI applications inevitably become more and more involved in our daily lives.
What excites you most about being at Johns Hopkins Department of Computer Science?
Hopkins is a great place to do trustworthy ML/AI research. I like the collegial and collaborative environment here. I have the opportunity to bridge the theory and application gap in machine learning by collaborating with language, vision, and robotics experts. I can also connect with ethics, policy, and sociology researchers.
Finally, Hopkins provides me with a unique opportunity and rich resources for investigating machine learning for health, a particularly safe-critical domain requiring assured ML/AI.
What classes are you teaching?
I am teaching the Machine Learning class this semester. The class covers the basics of supervised learning, deep learning, human-centered machine learning, and graphic models. It’s designed for upper-level undergraduates and graduate students who want to take their first dive into the machine learning field.
I am also teaching a graduate class on Machine Learning for Trustworthy AI. It’s designed for graduate students who want to develop their knowledge and understanding of the current research frontiers in trustworthy ML. They will also gain guided research experience by doing projects in this domain.
Besides your work, what are some of your other hobbies and passions? Is there anything your students or colleagues would be surprised to know about you?
I love hiking, swimming and playing badminton. I also enjoy meditation and listening to podcasts. I have even considered starting a podcast someday… if I find the time!