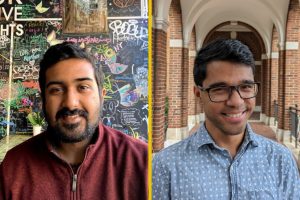
Two computer science graduate students have been named recipients of the Rising Stars Award, a program established by the Conference on Parsimony and Learning to highlight exceptional junior researchers at a critical inflection and starting point in their career.
CPAL is an annual research conference focused on addressing the parsimonious, low-dimensional structures that prevail in machine learning, signal processing, optimization, and beyond. Its Rising Stars Award program provides recipients with networking and mentoring opportunities and aims to increase representation and diversity within this field of computer science by providing a platform for awardees to present their research.
Ramchandran Muthukumar and Ambar Pal were chosen for exemplifying outstanding research potential by the CPAL community.
The Rising Stars will attend CPAL 2024 at the University of Hong Kong this week and present a short overview of their research in person.
Meet the Rising Stars
Ramchandran Muthukumar is a fifth-year PhD student advised by Jeremias Sulam. His research focuses on the theory of evaluating machine learning models and, in particular, evaluating the predictive performance of models on unseen and corrupted data. Central to Muthukumar’s work is the observation of local parsimony: Complex overparameterized models are equivalent to simpler models locally around each input. Effectively identifying and leveraging this local complexity has resulted in a fine-grained theoretical understanding of AI.
Muthukumar is from Chennai, India. He received a bachelor’s degree from the Birla Institute of Technology & Science, Pilani. He loves taking random walks, playing soccer, and spending time with his cat, Kiwi.
Ambar Pal is a final-year PhD student advised by René Vidal and Jeremias Sulam. His research focuses on the theory and practice of safety in artificial intelligence. Pal’s central philosophy is that incorporating structural constraints from data can efficiently mitigate current machine learning systems’ vulnerabilities to malicious agents. On the theoretical side, he has built frameworks formalizing how exploiting structure in data can lead to improved formal guarantees for safety. On the practical side, he has demonstrated the utility of such data-driven constraints for systems used for image and graph classification.
Pal is from New Delhi, India. He received a bachelor’s degree from the Indraprastha Institute of Information Technology, Delhi and a master’s degree from Johns Hopkins. In his spare time, he enjoys biking and studying microscopic organisms.